What is the significance of this specific data set? A crucial data collection, vital for analysis and insightful understanding.
The term "fsdss" likely refers to a specific dataset, potentially a file or collection of files. Without further context, it is impossible to precisely define its content or format. It might contain numerical, textual, or mixed data points, depending on the intended application. For example, it could be a dataset of financial transactions, sensor readings, or survey responses. Its precise characteristics are unknown without additional information. Understanding its structure and data types is crucial for effective analysis.
The value of such a dataset hinges on its potential for generating insights, revealing patterns, and supporting decision-making. Applications might include forecasting trends, identifying anomalies, or personalizing experiences. The historical context of its creation and usage would be informative for evaluating the dataset's utility and limitations. For example, data collection methodology would inform how dependable the findings are.
Read also:How To Fix Ssh Raspberry Pi Behind Firewall Not Working
Moving forward, a clear understanding of the data within this dataset is essential to determine its applicability and value in various contexts.
fsdss
Understanding the essential aspects of "fsdss" is crucial for effective analysis. Its characteristics dictate its potential applications and limitations. This exploration highlights key components to guide further investigation.
- Data collection
- Data structure
- Analysis methods
- Contextual relevance
- Potential biases
- Historical context
- Interpretation protocols
The seven aspects highlight the multifaceted nature of "fsdss." Data collection methods determine data quality, and structure influences analysis approaches. Contextual relevance and potential biases affect interpretation. Historical context shapes current data usefulness. Rigorous interpretation protocols ensure accurate findings, crucial for applications like trend forecasting. For instance, an historical dataset of financial transactions might reveal patterns of economic fluctuation, provided the data is structured and analyzed appropriately, without introducing biases from missing data or unreliable recording methods.
1. Data collection
Data collection forms the bedrock of any meaningful analysis. For "fsdss," the methodology employed in gathering the data is paramount. The quality and comprehensiveness of the data collection process directly affect the reliability and validity of subsequent analyses.
- Methodologies
Diverse approaches exist for data collection, each with strengths and weaknesses. Survey design, sensor deployment, or transactional record extractionthe specific method chosen significantly influences the dataset's characteristics and limitations. For instance, a poorly designed survey can introduce bias, while incomplete sensor readings might omit crucial data points. The appropriate methodologies should be carefully considered within the context of "fsdss" to maximize its analytical value.
- Data Sources
The origins of the data directly impact the dataset's integrity. Reliable and accurate data sources are crucial for producing trustworthy analyses. Data drawn from fragmented or inconsistent sources can lead to inaccurate conclusions. Examples include the difference between data from a publicly accessible database and data collected from a private company's proprietary system or data points manually recorded by different people at varied times.
Read also:
- Batman Tom Hardy The Dark Knights Legacy And Potential Future
- Sample Selection
Choosing a representative sample is critical for generalizability. A biased or unrepresentative sample can lead to erroneous conclusions. If the sample underrepresents a significant portion of the population, the conclusions drawn about "fsdss" will have limited applicability. Careful consideration of sampling parameters is vital for ensuring the analysis accurately reflects the entire dataset. Examples include stratified sampling, random sampling, and cluster sampling.
- Data Validation and Cleaning
Ensuring data accuracy is paramount. Errors, inconsistencies, or missing values can severely skew results. Validation and cleaning procedures must be carefully implemented to identify and address such issues. This process aims to maximize the dataset's value and minimize potential biases. Manual review, statistical analysis, and data quality assessment tools may be used for this purpose.
The aforementioned facets of data collection form an integral part of understanding "fsdss." Without careful consideration of these aspects, any subsequent analyses will be undermined. A comprehensive understanding of these practices, from method selection to data cleaning, is essential to extracting meaningful insights from the dataset and informing decision-making based on valid findings.
2. Data Structure
The structure of a dataset significantly influences its usability and analytical potential. For "fsdss," the format in which data is organized dictates how effectively it can be processed, analyzed, and interpreted. Understanding the specific structure of "fsdss" is crucial for determining appropriate analytical methods and ensuring accurate results.
- Data Types
The types of data within "fsdss" (e.g., numerical, categorical, textual) directly impact the applicable statistical analyses. Numerical data allows for calculations like averages and correlations; categorical data facilitates comparisons and classifications; textual data requires specialized natural language processing techniques. Recognizing the data types allows the selection of appropriate methods, avoiding misapplication of algorithms that are suited for one data type to another.
- Relational Structure
If "fsdss" involves multiple tables or interconnected datasets, understanding the relationships between them is crucial. Relational databases, for example, link data points across tables, facilitating sophisticated queries and analyses. Knowing the relationships allows for a holistic view and the linking of relevant data points for analysis, rather than treating isolated pieces of information. An example is a database linking customers to their purchase history.
- Data Format
The specific format of "fsdss" (e.g., CSV, JSON, SQL) dictates the tools and approaches available for processing. Choosing appropriate tools and techniques for data transformation, cleaning, and analysis depends on the chosen file format. CSV files are simpler but may require more manual cleaning, while JSON allows for richer and more structured data, potentially speeding up analysis.
- Data Granularity
The level of detail contained within "fsdss" affects the scope and precision of analysis. Highly granular data enables detailed examinations of specific trends and patterns, while aggregated data reveals broader, more general patterns. Understanding the granularity clarifies the appropriate level of detail required for the intended analysis. For instance, daily sales figures provide a higher level of granularity than monthly sales totals.
The data structure of "fsdss" is not merely a technical aspect but fundamentally shapes the potential insights extractable from the dataset. Careful consideration of the data types, relationships, format, and granularity allows for the application of correct methods, leading to meaningful results. This, in turn, will maximize the value of "fsdss" for its intended application.
3. Analysis Methods
The efficacy of any dataset hinges critically on the analytical methods applied. For "fsdss," the choice and application of appropriate analysis methods determine the quality and depth of insights derived. Effective methods translate raw data into actionable knowledge, while inappropriate methods can lead to misleading conclusions. The selection of analysis methods must be directly aligned with the characteristics of "fsdss" and the intended goals of the analysis, acknowledging that the data's inherent structure dictates the suitable methodologies.
Examples illustrate the importance of appropriate analysis. Consider a dataset of customer purchasing patterns ("fsdss"). Descriptive statistics (mean, median, standard deviation) could reveal average spending and purchasing frequency. Regression analysis might uncover correlations between demographics and purchasing habits. Clustering could identify distinct customer segments based on purchasing behavior. Each method provides a different facet of understanding, and the appropriate combination of methods yields a richer and more comprehensive portrait. In contrast, applying a time series analysis to customer demographic data would likely be inappropriate and yield irrelevant results. The crucial link lies in matching method to data type and question. The successful utilization of "fsdss" depends entirely on this understanding. Choosing the incorrect analysis method can lead to flawed results, which can be detrimental in decision-making, especially in areas such as marketing, finance, and healthcare. Selecting the right analytical methods for "fsdss" is not merely a technical exercise but a strategic decision that impacts interpretations and their subsequent applications.
In conclusion, the selection of analytical methods is integral to the value and utility of "fsdss." Appropriate methods extract meaningful patterns and insights from the data, whereas inappropriate methods can obscure rather than illuminate. The key takeaway is a direct link between the characteristics of "fsdss" and the suitability of chosen analysis methods. Understanding this relationship allows for informed decisions regarding data analysis, facilitating the extraction of actionable knowledge from raw data and, ultimately, promoting effective decision-making based on reliable interpretations.
4. Contextual Relevance
The significance of "fsdss" hinges on its contextual relevance. Meaningful interpretation of data within "fsdss" requires understanding the environment in which it was generated and the goals it was intended to serve. Without this context, patterns and insights derived from "fsdss" may be misconstrued or irrelevant. The specific setting, purpose, and methodology behind the data collection are paramount.
- Historical Context
Historical circumstances surrounding the creation of "fsdss" significantly impact interpretation. Economic trends, societal shifts, or technological advancements at the time of data collection influence the meaning and validity of patterns observed in "fsdss." For instance, comparing consumer spending data from the 1950s to the present day reveals differing economic realities. Recognizing this history avoids misinterpreting contemporary patterns as universal truths.
- Geographical Location
Geographic location influences the characteristics of data within "fsdss." Data collected in one region may exhibit distinct characteristics compared to data from another. Cultural norms, economic conditions, and environmental factors all play a role. For example, transportation patterns in a densely populated urban center will differ from those in a rural, sparsely populated area. Understanding the geographic location provides a crucial frame of reference for interpretation.
- Purpose of Data Collection
The intended purpose of collecting "fsdss" dictates the appropriate analyses. Data intended for forecasting economic trends will be analyzed differently from data collected to study customer preferences. Knowing the purpose allows for selecting relevant analytical tools. For instance, interpreting data from a public opinion poll designed to gauge public sentiment regarding a specific policy will differ from evaluating data gathered to track website traffic.
- Methodology of Data Collection
The methodology used to collect "fsdss" impacts the validity and reliability of the dataset. Biased sampling methods, inconsistent data entry, or inadequate survey design can undermine conclusions derived from "fsdss." Methods must be scrutinized to ensure data quality. For example, a market research survey targeting a specific demographic will provide limited insight into the entire market. Understanding the limitations due to the collection methodology helps in properly interpreting the findings.
In summary, contextual relevance ensures accurate interpretation of "fsdss." Ignoring the environment surrounding data collection can lead to erroneous conclusions. Careful consideration of historical background, geographic location, intended purpose, and data collection methodology enhances the reliability and value of any analysis derived from "fsdss," facilitating informed decision-making in various fields, from market analysis to policy formulation.
5. Potential Biases
Bias in datasets, such as "fsdss," represents a significant concern. Biases inherent in data collection, sampling, or analysis can lead to skewed interpretations and potentially inaccurate conclusions. Understanding these potential biases is crucial for evaluating the reliability and validity of findings derived from "fsdss." Identifying and mitigating biases is paramount to ensuring trustworthy insights. For example, a survey on consumer preferences, if targeted only at individuals from a specific socioeconomic background, will yield skewed results about the broader population's preferences. This skewed data would lead to inaccurate projections or marketing campaigns.
Several types of bias can affect "fsdss." Sampling bias arises from non-representative samples; for instance, a survey focusing only on a particular age group will not reflect the views of the entire population. Measurement bias arises when the measuring instrument or process systematically misrepresents the true value. Selection bias occurs when individuals are more likely to be included in the data due to certain characteristics. Observer bias results from the individual collecting data influencing the data, perhaps by consciously or subconsciously selecting specific information or interpreting data subjectively. Furthermore, confirmation bias, where individuals tend to favor information that confirms pre-existing beliefs, can influence the selection and interpretation of data. Each type of bias can lead to distorted conclusions. In the context of "fsdss," these biases need careful identification to understand and mitigate their impact.
The practical significance of understanding potential biases in "fsdss" is profound. For instance, inaccurate market research conclusions based on biased data can lead to flawed product development strategies or misdirected marketing campaigns. In clinical trials, biased data can lead to an overestimation or underestimation of drug effectiveness, with potentially severe consequences. Analyzing data from financial markets with hidden biases could lead to making poor investment choices. By acknowledging the existence and potential impact of various biases, appropriate measures can be implemented, such as employing diverse sampling techniques, rigorous quality control measures, and ensuring transparency in data collection and analysis, leading to more reliable and accurate conclusions. These steps promote trust in the findings derived from "fsdss." Ultimately, awareness of and attention to potential biases in "fsdss" is essential for effective decision-making in any field that relies on data-driven insights.
6. Historical Context
Historical context is inextricably linked to the understanding and interpretation of any dataset, including "fsdss." The circumstances surrounding the creation and collection of data significantly influence its meaning and validity. Historical events, social norms, economic conditions, and technological advancements all shape the data. These factors often act as underlying causes influencing the characteristics of the data, potentially creating bias or limitations. For example, census data from the early 20th century may reflect societal prejudices or limited recording methodologies that are absent in contemporary records.
Considering historical context becomes crucial for accurate interpretation and informed decision-making. Analyzing historical data within "fsdss" requires acknowledging the different societal and technological landscapes from which the data originated. Changes in measurement standards, evolving definitions of variables, and differing methodologies over time demand careful consideration. Understanding these historical factors allows researchers to account for potential biases and limitations, avoiding inaccurate generalizations and ensuring meaningful insights. For instance, if "fsdss" contains financial data from a specific period, knowledge of economic policies, regulatory changes, or major market events is crucial for contextualizing the data and understanding the underlying trends. Failing to account for such historical factors can misinterpret present-day situations, resulting in erroneous conclusions or inaccurate forecasts.
In conclusion, neglecting historical context when analyzing "fsdss" can lead to flawed interpretations. A deep understanding of the historical factors surrounding data collection and the dataset's origin is essential for proper evaluation and meaningful analysis. By acknowledging the past influences, researchers can extract more accurate insights, avoid erroneous conclusions, and apply the findings of "fsdss" more effectively and reliably in various fields. This rigorous approach allows for a deeper comprehension of the data and ensures a more nuanced perspective on its implications.
7. Interpretation Protocols
Interpretation protocols are essential for extracting meaningful insights from datasets like "fsdss." These protocols establish standardized procedures for handling and interpreting data, thereby ensuring consistency and minimizing bias in analysis. Without well-defined protocols, subjective interpretations can lead to misleading conclusions, undermining the reliability and value of the dataset.
- Clear Definitions and Operationalizations
Interpretation protocols should clearly define terms and concepts used within "fsdss." For example, if "fsdss" contains survey data about consumer preferences, precise definitions for terms like "high satisfaction" or "frequent buyer" must be established. Operationalizing these terms into measurable criteria ensures consistency in data interpretation, avoiding ambiguities. Without these specific, measurable definitions, interpretations vary, potentially leading to contradicting conclusions. This is crucial for datasets with potentially subjective elements, or where prior understandings may be inaccurate or misleading.
- Established Standards for Data Validation
Protocols must incorporate rigorous data validation standards. Procedures for identifying and addressing inconsistencies, errors, or missing values within "fsdss" must be explicitly stated. For example, protocols should detail steps for checking data entry accuracy, identifying outliers, and handling missing data points. Consistent application of these standards assures data quality, a critical factor in avoiding misleading interpretations of patterns and relationships within "fsdss." Failing to address data validation thoroughly can lead to inaccuracies in subsequent analyses, making the insights unreliable.
- Standardized Analytical Techniques
Protocols should prescribe specific analytical techniques and statistical methods. For example, if "fsdss" contains financial data, protocols should specify whether to use descriptive statistics, regression analysis, or time series modeling. Standardizing these methods ensures comparability across different analyses and minimizes subjectivity in drawing conclusions. Consistent use of statistical methods minimizes bias and improves reliability in evaluating data patterns. Inconsistency in analysis methods produces differing interpretations, making valid comparisons challenging.
- Documentation of Procedures and Assumptions
Thorough documentation is crucial. The protocols must clearly outline all steps involved in data interpretation. Any assumptions made during the analysis must be explicitly stated. This transparency allows for reproducibility and scrutiny by other researchers. This documentation facilitates a thorough understanding of the reasoning behind conclusions drawn from "fsdss." Lack of documentation obscures the processes used in analysis, potentially hindering reproducibility and review.
Adherence to well-defined interpretation protocols is paramount for the reliability and value of datasets like "fsdss." By establishing clear definitions, rigorous validation procedures, standardized analytical techniques, and comprehensive documentation, analysts can ensure consistent and objective interpretations, leading to more robust and trustworthy insights.
Frequently Asked Questions about "fsdss"
This section addresses common inquiries regarding "fsdss," aiming to clarify key aspects and dispel potential misconceptions. A comprehensive understanding of "fsdss" is crucial for utilizing the dataset effectively.
Question 1: What does "fsdss" stand for?
The precise meaning of "fsdss" is unclear without additional context. It likely refers to a specific dataset but, without further information, cannot be definitively defined.
Question 2: What types of data are typically found within "fsdss"?
The nature of the data within "fsdss" is indeterminate without additional information. It could include numerical, textual, or mixed data, depending on the source and intended use.
Question 3: What are the potential applications of "fsdss"?
The specific uses of "fsdss" depend on its content and structure. Potential applications span diverse fields, including forecasting trends, identifying patterns, and supporting decision-making processes.
Question 4: How can I obtain the "fsdss" dataset?
Accessing "fsdss" requires knowledge of its origin and intended distribution channels. Contacting the source or custodian of the data is typically necessary. Without additional details, obtaining the dataset is impossible.
Question 5: What are the limitations of using "fsdss"?
Limitations of "fsdss" are dependent on its characteristics, including data quality, potential biases, and historical context. Uncritical reliance on the data may produce inaccurate conclusions without careful consideration of potential drawbacks.
In summary, a clear understanding of the specific dataset, including its nature, source, and intended use, is vital for appropriate interpretation. Without this comprehensive context, drawing reliable conclusions from "fsdss" is not possible. Further investigation, including seeking additional details about the dataset's origins and intended application, is recommended.
The following section delves into detailed considerations regarding data collection, structure, and analytical methods applicable to datasets such as "fsdss."
Conclusion
The exploration of "fsdss" highlights the critical need for thorough investigation before analysis. Without a clear understanding of the dataset's origins, structure, and intended use, any conclusions drawn are inherently unreliable. Key factors, including data collection methodologies, potential biases, and the historical context surrounding "fsdss," must be meticulously considered. Interpretative protocols, establishing standardized procedures for handling and interpreting the data, are essential to ensure accuracy and avoid misinterpretations. The significance of "fsdss" rests entirely on its contextual relevance. This analysis emphasizes the importance of responsible data handling and the critical role of meticulous investigation in deriving meaningful insights from any dataset.
Moving forward, researchers and analysts must prioritize a comprehensive understanding of datasets like "fsdss" before embarking on any form of analysis. This involves meticulous scrutiny of data quality, a clear articulation of analytical methods, and a profound appreciation for potential biases and limitations. By adhering to rigorous protocols and recognizing the interplay between data, context, and interpretation, more reliable and trustworthy findings can be generated. Furthermore, open communication, documentation, and collaboration across disciplines are crucial to achieving robust insights and informed decisions.
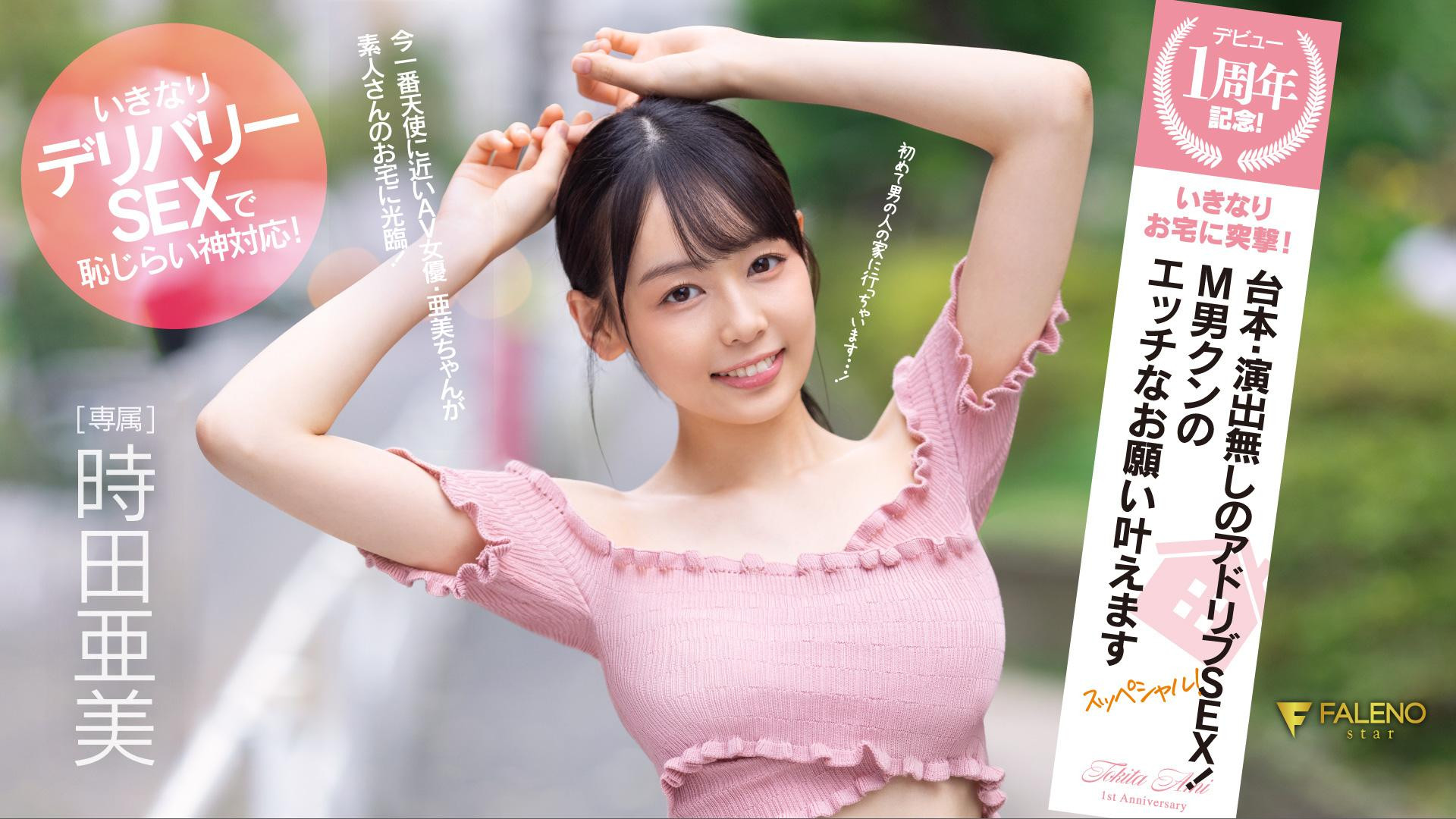

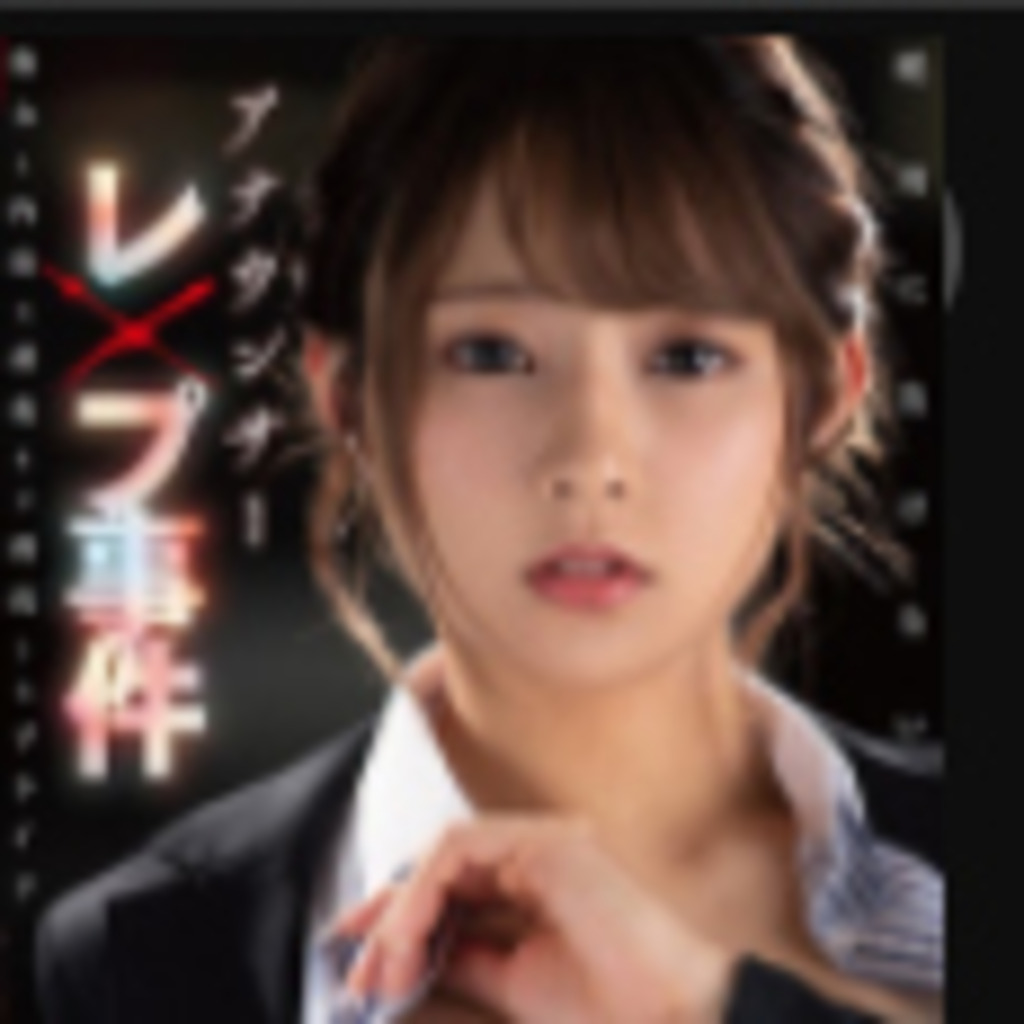